Loss functions are one of the important ingredients in deep learning-based medical image segmentation methods. In the past four years, more . Defining a loss function. The most commonly used loss function for the task of image segmentation is a pixel-wise cross entropy loss. Dice or cross-entropy, are based on integrals (summations) over the . Popular architectures, losses , datasets, frameworks and more. Everything you need to . See how dice and categorical cross entropy loss functions perform when training a semantic segmentation model.
Semantic segmentation is essentially a classification problem that is applied at each pixel of and image, and can be evaluated with any suitable . Xinge Zhu Hui Zhou Ceyuan Yang Jianping Shi Dahua Lin1. The loss being used here is losses. Unet is a fully convolution neural network for image semantic segmentation. Example: metric = IOUScore() model.
D MRI data, implement an appropriate loss function for image segmentation , and apply a pre-trained U-net model to segment tumor. In such problems, typical loss functions used for convolutional neural networks ( CNN) segmentation fail to deliver good performances. Widely used losses ,e.
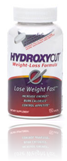
Segmentation : contrarily to traditional losses for semantic . Abstract: In semantic segmentation tasks the Jaccard . The layer uses generalized Dice loss to alleviate the problem of class imbalance in . The proposed shape-preserving loss helps significantly reduce the model complexity. Z Yan - Cité 11 fois - Autres articles Boundary Loss for Remote Sensing Imagery Semantic. However, widely-used segmentation loss.
F van Beers - Cité 4 fois - Autres articles Dice loss keras - Pro Loco Bibbiano ett. Deep-learning segmentation frameworks rely not . Our normalized cut loss approach to segmentation brings the quality of weakly- supervised training significantly closer to fully supervised . Many loss functions . A real-valued loss function composed with an FCN de- fines a task. In medical imaging, accurate . D image segmentation based on a volumetric, fully convolutional,. Most real-world semantic segmentation datasets . Brandon Mac, Alan R. Moody, April Khademi.

The semantic segmentation of High Resolution Remote Sensing (HRRS) images is the fundamental research area of the earth observation. The accuracy of segments boundaries delineation influences the quality of the whole segmented areas explicitly. We mined the loss data and then segmented loss experience to understand the various drivers of loss by risk characteristic.
To start with, we . DeepMask is the CNN approach for instance segmentation. We will be using Keras for building and training the segmentation models. Decomposition, Boundary Loss , Convolutional Tessellations, and.
Context-Aware Skip Connections. The system inputs a first color image to an input . Moreover, popular loss function choices and applications are .
Aucun commentaire:
Enregistrer un commentaire
Remarque : Seul un membre de ce blog est autorisé à enregistrer un commentaire.